Unlocking Data-Driven Insights in Finance: The Power of Text Analysis and NLP
In today’s data-driven world, financial organizations are constantly seeking ways to extract meaningful insights from the vast amounts of data at their disposal. One of the most valuable, yet often underutilized, data sources is unstructured text—emails, customer feedback, social media posts, and other forms of communication. With the advent of advanced text analysis and natural language processing (NLP) technologies, financial institutions can now leverage this data to improve decision-making, optimize processes, and enhance customer engagement.
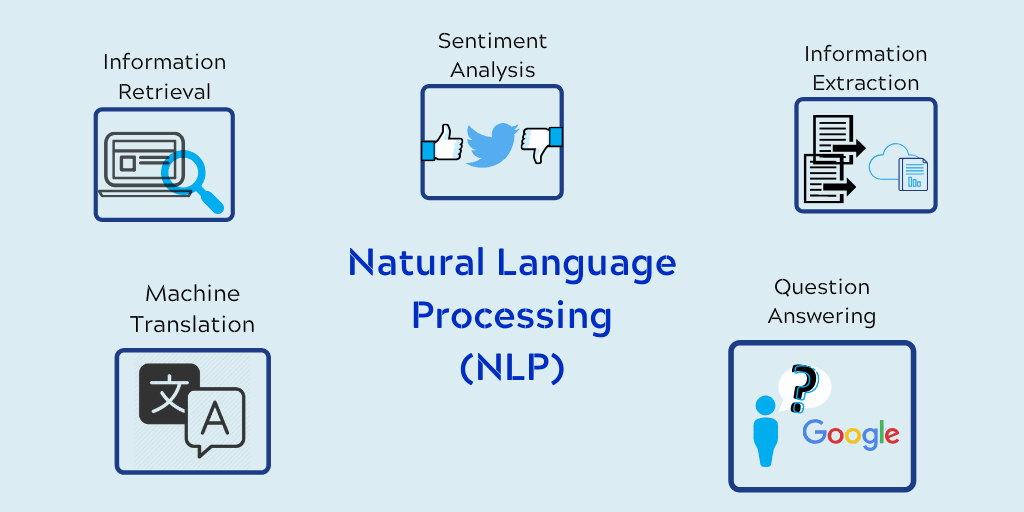
1. The Growing Need for Text Analysis in Finance
Financial organizations deal with enormous volumes of unstructured text every day, whether through customer inquiries, regulatory documents, news articles, or internal communications. Historically, extracting insights from such data required manual processes, which were time-consuming and error-prone. With the rise of text analysis and NLP, these institutions can now automate the interpretation of text data, allowing for quicker and more accurate decision-making.
For example, sentiment analysis—a technique enabled by NLP—can assess customer feedback, social media posts, and even call center transcripts to understand customer sentiment in real time. This not only helps financial institutions monitor their brand perception but also identifies potential risks or opportunities. Similarly, text classification tools can organize vast amounts of legal documents, contracts, and reports, ensuring that organizations stay compliant with regulatory requirements without overwhelming their legal teams.
2. NLP-Driven Insights for Customer Service and Experience
Customer service is one of the most critical areas where financial institutions benefit from NLP and text analysis. With NLP technologies, companies can now analyze customer queries, complaints, and requests with unprecedented accuracy and speed, leading to faster resolutions and improved customer satisfaction.
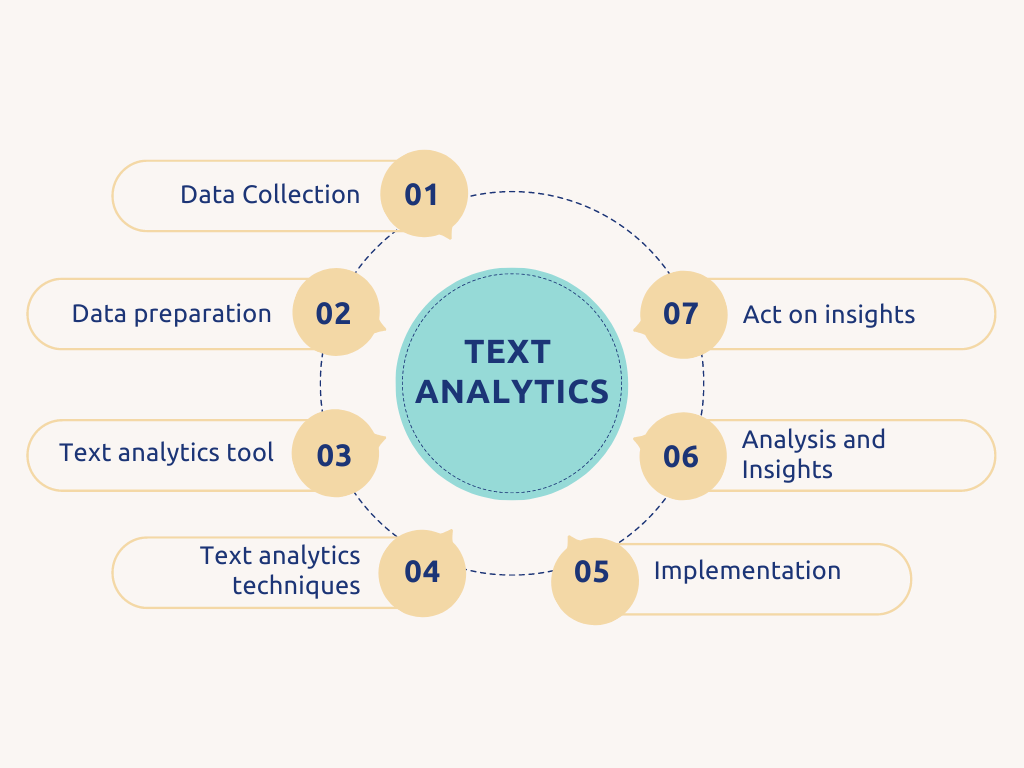
For instance, financial service providers can implement intelligent chatbots or virtual assistants powered by NLP to handle routine queries such as account balances, transaction statuses, or payment issues. These virtual assistants are capable of understanding context and intent, providing customers with the information they need almost instantly.
Beyond automation, text analysis allows financial institutions to segment and analyze customer feedback at scale, identifying recurring issues or concerns. This helps businesses prioritize customer needs and optimize their service delivery. In an industry where customer loyalty is paramount, offering responsive and personalized support through NLP-driven solutions can significantly improve client retention and brand reputation.
3. Enhancing Risk Management with Data-Driven Tools
Risk management remains a cornerstone of the financial sector, and text analysis can play a transformative role in this domain. Through the use of NLP, organizations can analyze financial reports, market news, and social media trends to detect early signals of risk, such as market volatility, regulatory changes, or emerging fraud schemes.
For example, NLP tools can sift through vast datasets, identifying patterns that human analysts may overlook. These technologies can flag potentially risky transactions, detect unusual account behavior, and assess sentiment in financial news or regulatory updates. By leveraging such insights, institutions can respond faster to emerging threats, thereby reducing exposure to financial loss.
Additionally, regulatory compliance and risk reporting can be significantly streamlined with text analysis. Automating the extraction and categorization of key information from legal texts, compliance documents, and financial reports ensures that financial institutions maintain their adherence to regulations while reducing operational bottlenecks.
4. Transforming Financial Decision-Making with Text Analytics
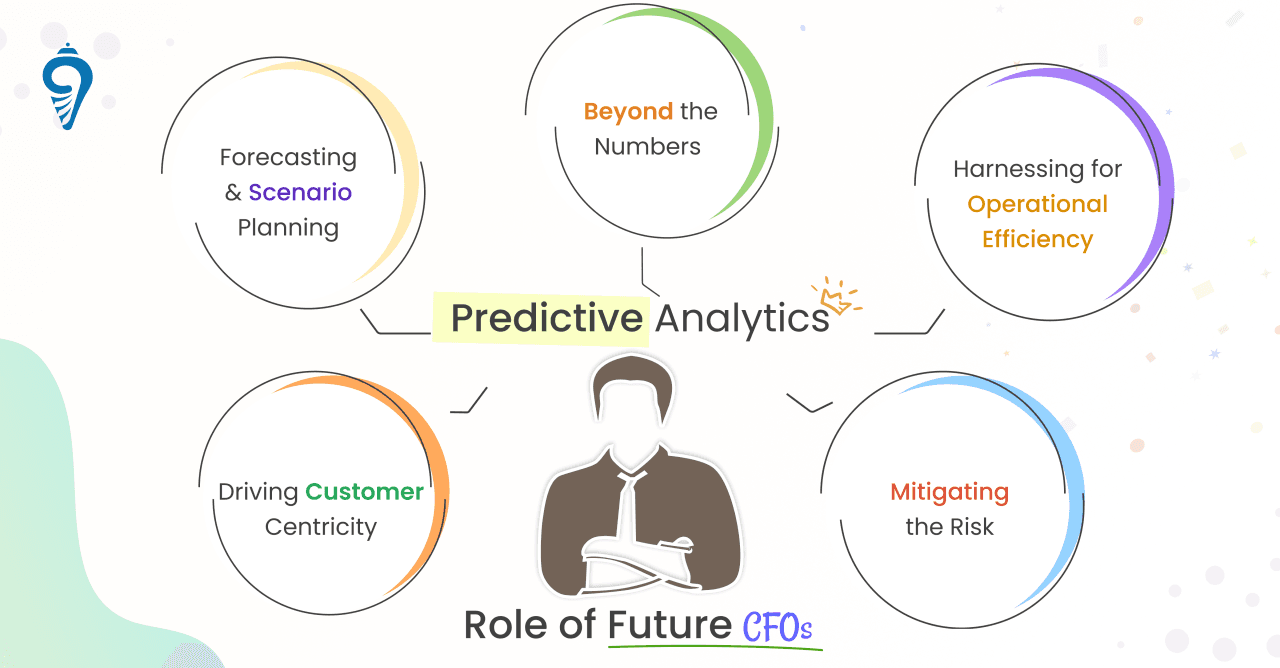
At the core of text analysis and NLP’s potential lies their ability to enhance data-driven decision-making. By tapping into unstructured data sources and integrating them with structured data analytics, financial organizations can make more informed, accurate, and timely decisions.
For example, in the context of investment strategies, text analysis can provide critical market sentiment data derived from earnings reports, CEO speeches, and global financial news. By understanding the emotional tone behind these communications, financial analysts can better predict market trends and adjust their portfolios accordingly.
In lending, credit scoring models can benefit from analyzing alternative data sources, such as social media or online reviews, using NLP to assess a borrower’s reputation or financial responsibility. This approach complements traditional financial metrics, enabling lenders to make more holistic and accurate lending decisions, particularly in underbanked or underserved markets.
Conclusion
The adoption of text analysis and NLP in financial organizations is no longer a luxury—it is becoming a necessity for staying competitive in an increasingly data-driven landscape. By harnessing the power of these technologies, financial institutions can improve their customer service, streamline risk management, and make more informed, data-driven decisions. For business leaders, the message is clear: the future of finance is deeply intertwined with the ability to unlock insights from the vast volumes of text data available.
Investing in advanced text analysis and NLP tools today will not only enhance operational efficiency but also ensure that your organization remains agile and responsive in a fast-evolving financial ecosystem. As data continues to grow, those who master its interpretation will lead the way in innovation, customer satisfaction, and sustainable growth.